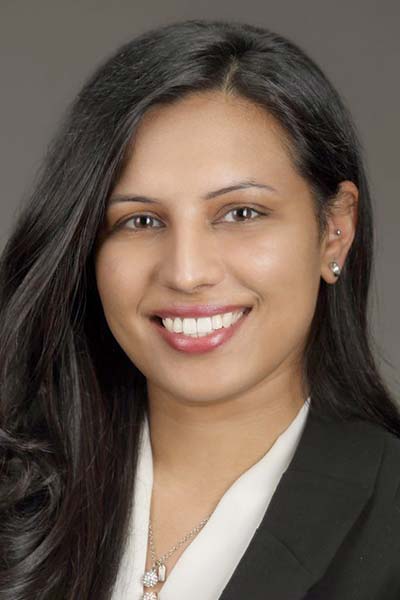
The role of artificial intelligence in healthcare continues to evolve—rapidly. ILCN’s ongoing series on the implications this technology may have on the diagnosis and treatment of lung cancer has highlighted both the opportunities and the challenges presented by AI. Chief among the concerns raised regarding the expanding role of AI in healthcare, are issues related to patient safety and equity. As the healthcare profession seeks to leverage AI to improve patient care, it will fall to regulators to ensure those concerns are adequately addressed.
ILCN recently spoke with the US Food and Drug Administration’s Oncology Division Director Harpreet Singh, MD, about the rapidly evolving role of AI in healthcare and what that means for regulating drug and device development. The interview has been edited for length and clarity.
ILCN: Is there a need to regulate the use of artificial intelligence in clinical research? How likely is it that AI could lead us to erroneous conclusions or false interpretations of the data, and is the FDA concerned about such possibilities?
Dr. Singh: As a general matter, the evidentiary standards needed to support investigational new drugs and/or drug approvals remain the same regardless of the tools or technological advances involved. The use of AI as part of the drug development process, including in clinical trials, should be described in the sponsor’s investigational new drug (IND) application and will be reviewed by the FDA in accordance with the applicable regulations.
FDA has received and reviewed hundreds of submissions for drug approvals since 2016 that included AI. These submissions spanned a range of therapeutic areas with oncology, psychiatry, gastroenterology, and neurology accounting for the largest numbers of AI-related submissions between 2016 and 2021. FDA recognizes the potential for AI to enhance drug development in many ways. AI might help bring safe and effective drugs to patients faster; provide broader access to drugs and thereby improve health equity; increase the quality of manufacturing; enhance drug safety; and help develop novel drugs and drug classes and personalized treatment approaches. The use of AI may result in increased diversity and representation (to the intended patient population) in clinical studies.
As with other evolving fields of science and technology, there are challenges associated with AI use in drug development, such as ethical and security considerations, including improper data sharing and cybersecurity risks. There are also concerns about using algorithms that have a degree of opacity, or algorithms that may have internal operations that are not visible to users or other interested parties. To address these concerns, in 2023, the FDA released a discussion paper, “Using Artificial Intelligence and Machine Learning in the Development of Drug and Biological Products,” to engage with various interested parties on these unique challenges. FDA will continue to provide regulatory clarity in this space and as part of its commitments under the Executive Order on the Safe, Secure, and Trustworthy Development and Use of Artificial Intelligence.
ILCN: Along these lines, AI algorithms are only as good as the data used to train them. Does the FDA have a plan to ensure that biases and inequities are not perpetuated in AI algorithms when the training data lack diversity?
Dr. Singh: During the review process, the FDA is engaged in identifying the possible risks associated with the use of AI, when applicable, and ensuring the sponsor has appropriately mitigated those risks. Sponsors should be transparent in the marketing applications about how the technology was developed and used. The context of use is essential in understanding and addressing possible bias from these data-driven tools.
ILCN: Another potential use for AI in research could be evaluating disease burden. To what extent do you think AI will facilitate RECIST/tumor burden assessments? Can it replace physician-delineated RECIST evaluations?
Dr. Singh: FDA recognizes the interest and potential for AI research tools to aid in disease evaluation, such as with RECIST measurements. FDA has authorized a number of radiological AI-enabled image analysis tools for clinical use, including tools to aid the evaluation of disease burden. Most tools are semi-automated, meaning that a physician can review and edit the measurements. The FDA issued guidance with recommendations for developers of tools to support quantitative image analysis.
ILCN: How is the FDA approaching the review and approval of devices, software, or other clinical tools that rely on artificial intelligence? How do you assess the safety and efficacy of AI-based devices? How is the FDA approaching bias in AI-based devices?
Dr. Singh: In addition to being committed to ensuring that drugs are safe and effective, including when AI is used in the development of those drugs, the FDA regulates AI-based software that meet the agency’s medical device definition. to ensure that they are safe and effective for the intended use and population. During premarket review, FDA subject matter experts assess the benefit/risk profile of a device for its proposed intended use and evaluate devices for their safety and effectiveness.
Healthcare delivery is known to vary by factors such as race, ethnicity, and socio-economic status; therefore, it is possible that biases present in our healthcare system may be inadvertently introduced into the algorithms. The agency recognizes the need for improved methodologies to identify and improve AI algorithms, including the identification and elimination of bias and initiatives to ensure the robustness and resilience of these algorithms to withstand changing clinical inputs and conditions. Advancing health equity for all devices is a top priority for the FDA. This aligns with the Biden Administration’s Executive Order on advancing racial equity and support for underserved communities. Thus, the FDA is actively assessing new policies, regulations, and guidance documents, which may be necessary to advance equity in agency actions and programs.
ILCN: What coordination or communication has there been between the FDA and other regulatory agencies worldwide to establish best practices for regulating the use of AI in healthcare?
Dr. Singh: The FDA actively engages in collaborative efforts with global regulators, developers, patient groups, academics, and other interested parties to establish best practices for regulating the use of AI in healthcare. This involves close cooperation with harmonizing bodies such as the ICH (International Council for Harmonization of Technical Requirements for Pharmaceuticals for Human Use) and the IMDRF (International Medical Device Regulators Forum). The FDA recognizes the importance of coordination and communication and aims to foster a responsive regulatory environment that addresses the unique challenges posed by AI in healthcare.
FDA continues its commitment to create a regulatory ecosystem that can facilitate AI innovation and adoption while safeguarding public health. For example, in 2021, the FDA, Health Canada, and the United Kingdom’s Medicines and Healthcare Products Regulatory Agency (MHRA) jointly published 10 guiding principles to inform the development of Good Machine Learning Practices (GMLP) for medical devices that use AI and machine learning.
The guiding principles touch on a wide range of issues, including the importance of bias mitigation, by ensuring that the results from machine learning models are generalizable to the intended patient population, and that the underlying datasets used to train these models are representative of the target population.
The principles also underscore the importance of safety and performance monitoring as algorithms evolve after being deployed in the “real world.” Specifically, controls should be in place to manage risks of overfitting, unintended bias, or degradation of the model that may negatively impact the safety and performance of the machine learning algorithm.
Data governance and transparency are also highlighted. Specifically, the GMLPs emphasize that the AI development process should include a “human in the loop,” where an expert human guides the cyclical development of the machine learning algorithm; it should all include end-users (i.e., providers and or patients) who are provided access to clear and contextually relevant information. These principles are now a work item for the IMDRF to harmonize globally.
ILCN: Finally, what is your biggest concern regarding the use of AI in the practice of oncology?
Dr. Singh: Thanks for the question! I don’t have concerns per se, but I do think we are very early in the process of establishing AI as a reliable tool for the practice of oncology. Many of the questions posed here get at some of the challenges and opportunities we are likely to encounter. I think AI can be an excellent tool to help augment the practice of oncology, but right now we are still learning its capabilities and limitations. Thanks again for having me!