Editor’s Note: This is Part 1 of a 2-part article shedding light on AI use in thoracic oncology. Part 1 explores the basics of AI and how it is being used today. In Part 2, Dr. Mak and Mr. Haugg discuss what the future may hold for AI in lung cancer. For even more from ILCN’s in-depth look at AI in thoracic oncology, see our patient perspective from patient advocate Angus Pratt.
Since ChatGPT emerged, artificial intelligence (AI) has received increasing attention. From the automation of drafting emails, screenplays, programming, coding, and more to AI-generated art and autonomous driving, the breadth and depth of possibilities of AI now seem limitless.
Though many may not recognize it, most of us interact with AI daily. This can range from recommendation systems for products on online shopping platforms, social media, and streaming services to facial recognition and voice assistants. As for medical applications, AI has many potential uses, including health screening, diagnosing diseases, predicting patient outcomes, personalizing treatment plans, and even facilitating drug discovery.
In this context, we turn the spotlight onto the field of oncology, specifically thoracic oncology, to examine how AI is currently being harnessed and offer insights into the exciting possibilities that lie ahead.
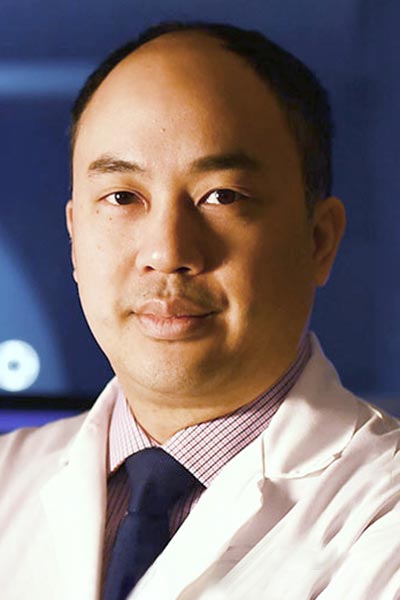
What Is AI?
An encompassing definition characterizes AI as replicating human intelligence by machines, particularly computer systems, to enable enhanced problem-solving.1 The term is frequently used interchangeably with its subdomains, such as machine learning and deep learning, which are specialized areas within AI.
Two common branches of AI are computer vision and natural language processing, with the former focusing on understanding objects and people in images and videos—interpreting the visual world. The latter, natural language processing, focuses on interpreting written or spoken text, and enables computers to understand, generate, and respond to human language.
Regarding underlying methodologies, machine learning is a computational approach designed to learn from data by identifying underlying rules and patterns for data processing.2 Compared to deep learning, machine learning depends more on human input for training the algorithm. An expert determines which features are to be learned, meaning the input data requires more structure and curation.
On the other hand, deep learning—a subfield of machine learning—uses methods that mimic the neural architecture of the human brain by structuring algorithms in layers called artificial neural networks, while leveraging advances in computational power and massive data sets. Although simpler machine learning algorithms can decipher patterns that humans can more easily understand, the inner workings of deep learning algorithms may not be as easily interpretable and can seem like a “black box.”3
Deep learning operates in a way that makes it challenging to understand the internal mechanisms and decision-making processes behind its predictions or classifications. This is primarily due to the numerous layers of connected nodes, or “neurons,” that process input data. Each layer of the network transforms the data, making the exact decision path increasingly opaque as the data moves deeper into the network. In this case, we can assess only the input and output of the model, with the intermediate process remaining opaque.
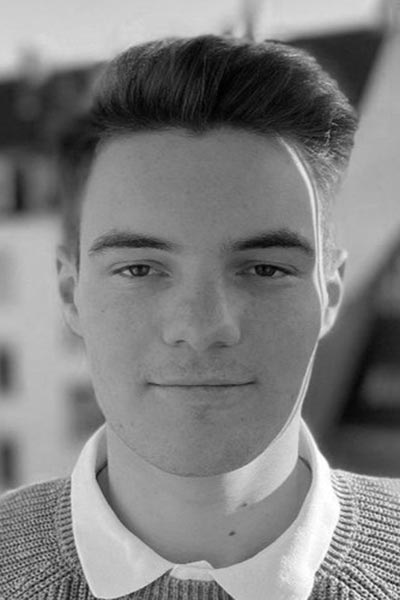
To illustrate at a high level without technical complexity, let’s consider a classification task of identifying a cat versus a dog from a photograph, which is a seemingly trivial task for humans, but a more difficult challenge for machines. The human programmer could train a traditional machine learning algorithm to recognize cat-like features, such as longer whiskers or eye and ear shapes, which would yield false positive and negative results. In comparison, a supervised deep learning training approach might involve a human labeling thousands of different images as either cat or dog and then training a convolutional neural network on this massive data to perform this classification. The human end user might not know the exact features that allow the deep learning algorithm to make this classification.
Despite this complexity and black-box nature, deep learning’s power to synthesize highly complex and massive amounts of data has been clearly illustrated. One notable example are generative models, such as ChatGPT. A generative model is trained to generate new data that resemble the data the model is trained on, rather than just generating a prediction.
For example, ChatGPT is a large language model trained on vast amounts of text data that can generate human-like text, most likely in context to the input it received. ChatGPT was trained on a diverse mix of datasets, including a dataset known as Common Crawl,4 which alone contains nearly a trillion words before filtering. It is worth noting that this model learns in an unsupervised manner—it does not rely on labeled data; instead, it learns the probability distribution of the training data—it predicts the next word in a sentence based on probabilities it has learned from all the training text, and then predicts the following sentence and so on.
Unsupervised deep learning algorithms are designed to analyze and classify unlabeled datasets. They discern underlying patterns and structures without explicit human instruction, learning purely from the data’s inherent structure and relationships. This approach is central to the concept of foundation models. Instead of training individual deep learning algorithms for a specific task—which requires a large amount of task-specific data, is subject to over-fitting, and is prone to bias—foundation models leverage self-supervised learning approaches to train task-agnostic models first and then use transfer learning to tailor to a specific task.
Suppose we employ an unsupervised learning model on the cat and dog image classification problem. Without any pre-set categories, the model might cluster the images by animal type—cats in one group, dogs in another—based on distinct underlying features. However, the model is encoded with all the intrinsic features observed in the photographs so that an alternative output could see the model clustering based on size—grouping larger and smaller animals, regardless of species. This exemplifies the flexible, data-driven nature of unsupervised learning models.
Lastly, this example can provide insights into the concept of generative AI models, where the encoded data within the model can then be used to generate new content. In the cat and dog example, a generative AI model can be used to generate new images of cats and dogs that are synthesized from all the images encoded in the model. Clearly, the ability of these approaches to generate new data and insights from synthesizing massive amounts of data will have important applications in medicine, such as synthesizing clinical data for risk predictions or new targets in drug discovery.
Where Are We Now In Oncology?
The impact of AI on oncology will be significant. These early innovations have primarily been directed toward improving prediction models and diagnostic accuracy. For instance, several significant studies have leveraged machine learning to predict mortality rates in cancer patients using structured electronic health record (EHR) data.5,6 These models have successfully integrated into EHR systems, demonstrating real-time, accurate predictions of short-term mortality for patients with cancer. The vast data available within EHR systems showcase the untapped potential for AI.
More advanced deep learning techniques have also shown promise in clinical applications to unstructured EHR text data and medical imaging through natural language processing and computer vision approaches, respectively. For example, deep learning algorithms can be trained on CT scans of patients with lung cancer that were labeled by clinician experts, and these algorithms have demonstrated an ability to identify and segment lung tumors with an accuracy and efficiency that matches manual delineation by medical professionals.7
Moreover, FDA approval for several AI-based radiology devices with a variety of use cases, including computer vision applications for enhanced radiological diagnosis or segmentation, further illustrates the rapid clinical implementation of these technologies in healthcare.8 These advancements in AI will play a transformative role in oncology, setting the stage for more personalized, efficient, and precise cancer care.
How Can AI Be Applied For Lung Cancer Care?
Next, we will highlight some of the most promising applications of AI in thoracic oncology and contextualize their use in the framework of a lung cancer patient’s clinical journey, visualized in Figure 1.
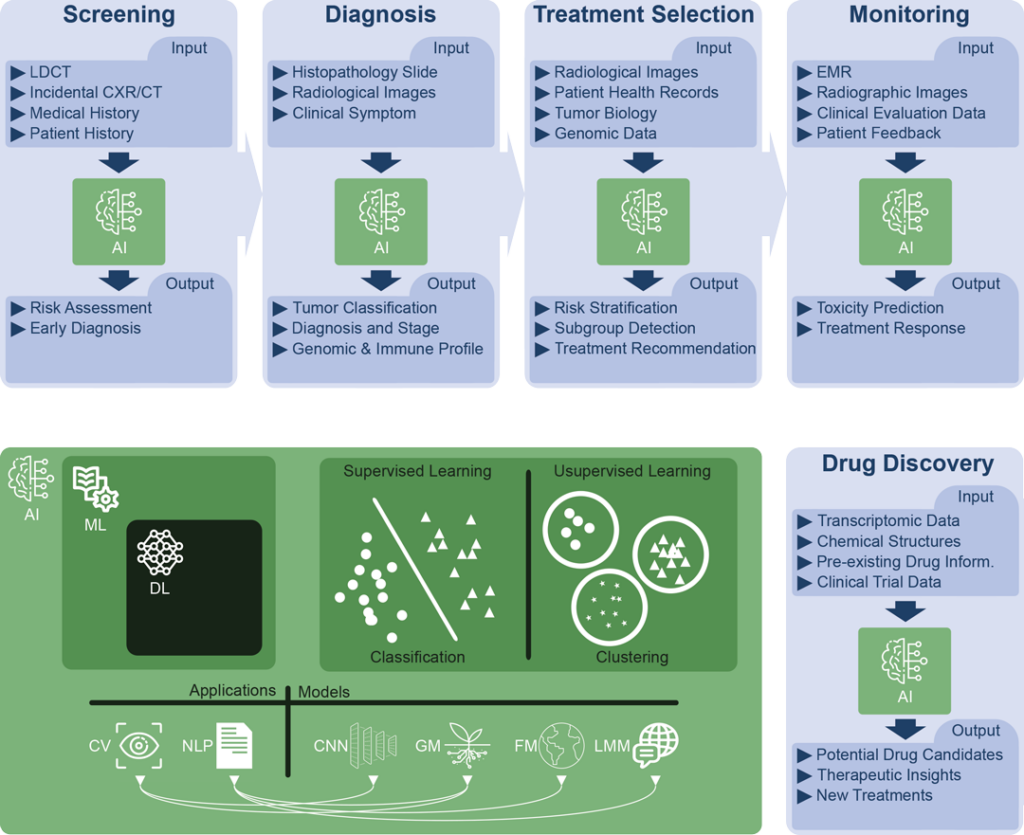
Fig. 1. This visualization provides an illustrative exploration of AI applications and their significant roles across the lung cancer patient journey and in research and drug discovery. Simultaneously, the graphic highlights the interconnected subdivisions of AI, represented in green, and their respective definitions:
- Artificial Intelligence (AI): AI encompasses the broad field of creating machines capable of intelligent behavior.
- Machine Learning (ML): ML is a subdomain of AI that uses algorithms to identify patterns in data and make predictions or decisions based on prior data patterns without being explicitly programmed to perform the task.
- Deep Learning (DL): A subdomain of ML, DL employs multi-layered artificial neural networks to understand complex patterns in large amounts of data, driving advancements in fields like natural language processing and computer vision.
- Applications
- Natural Language Processing (NLP): NLP, a subfield of AI, studies interactions between computers and human language, focusing on enabling computers to understand, interpret, and generate text.
- Computer Vision (CV): CV, another subfield of AI, focuses on equipping machines to interpret and understand visual data from the surrounding world.
- Models
- Generative Models (GM): These are statistical models in ML and DL that learn the data distribution of the training set to generate new data points, and they play significant roles in both NLP and CV tasks.
- Foundation Models (FM): Foundation Models are a class of AI models, including large language models, that serve as a general-purpose base for a wide range of downstream tasks, leveraging supervised and unsupervised learning approaches to gain proficiency across fields such as NLP and CV.
- Large Language Models (LLM): LLM are AI models that, through DL techniques and generative models, have been trained on extensive amounts of text data to understand and generate human language, enhancing capabilities in NLP.
- Convolutional Neural Network (CNN): CNNs are a specialized type of artificial neural network model used primarily for computer vision tasks, adept at processing grid-like data (e.g., images) and detecting spatial features.
- Model Training Approaches
- Supervised Learning: Supervised learning is a training paradigm in ML and DL where models learn patterns from labeled data to make predictions or decisions.
- Unsupervised Learning: Unsupervised learning is an approach in ML and DL in which models learn to identify patterns and structures from unlabeled data.
- Model: In the context of AI, a “model” is an algorithm that has been trained on data to identify patterns, and it is used to make predictions or decisions about new or unseen data
Acronyms:
CT = Computed Tomography, CRX = Chest Radiograph, Inform. = Information, LCDT = Low-Dose Computed Tomography, Resp. = Response.
Let us begin with lung cancer screening. In this stage, multiple AI models have been shown to augment CT screening, for instance, with the development of Sybil,9 a deep learning model, which predicts an individual’s future risk of lung cancer from a single low-dose CT (LDCT) scan. Furthermore, deep learning algorithms like CXR-LC by Lu et al.10 have been developed to predict lung cancer incidence using patient data such as age, sex, and smoking status, alongside a chest radiograph. The resulting model was validated in two large lung cancer screening trials and found to have a better discrimination rate for incident lung cancer than the standard Center of Medicare eligibility criteria, demonstrating its potential as a more effective tool for lung cancer screening.
Next, at the time a lung cancer diagnosis is made, digital pathology, an emerging field for AI applications, promises to enhance the accuracy and speed of diagnostic processes. For example, Coudray et al.11 have presented an approach using a convolutional neural network to classify lung tumor histopathology accurately into adenocarcinoma, squamous cell carcinoma, or normal lung tissue. The deep learning model achieved high accuracy in classifying lung tumor histopathology slides into different subtypes, demonstrating comparable performance to pathologists and the potential to predict the presence of common gene mutations (e.g., EGFR, KRAS, STK11, and TP53) from whole-slide images, offering a promising tool for accurate diagnosis and personalized treatment decisions in lung cancer.
Pathologic diagnosis can also be supported by quantitative radiologic (aka radiomics) analyses. For instance, Chaunzwa et al.12 used deep learning techniques to predict the histology of non-small cell lung cancer (NSCLC) tumors using CT images, and other studies have demonstrated the ability of deep learning algorithms to predict tumor genomics and immune biomarker expression from radiological images of the tumor.13 If these non-invasive approaches prove to be capable of enhancing diagnostic accuracy, they could improve the personalization of treatment selection, and provide serial, non-invasive imaging of biomarkers of tumor evolution during and after treatment.
Following diagnosis, the machine learning algorithm may inform treatment selection, especially in assessing patient risk profiles. For instance, Xu et al.14 used unsupervised learning to categorize older adults with advanced cancer into clusters of low, moderate, and high symptom severity. Their findings showed that patients with higher symptom severity at the onset of treatment were more prone to unplanned hospitalization and had poorer survival outcomes. This underscores the potential of machine learning in healthcare for identifying patient cohorts at elevated risk of adverse outcomes. Consequently, this could assist clinicians in making more informed treatment decisions by providing a nuanced understanding of patient risk profiles.
Additionally, AI has the potential to automate and improve treatment response assessment. For example, natural language processing has been studied as a means to automatically extract clinical outcomes from radiology reports and oncologist notes in electronic health records.15,16 Moreover, deep learning computer vision applications to serial CT scans in follow-up after therapy may improve our ability to predict clinical outcomes and treatment response in patients with locally advanced NSCLC, as evidenced by the study conducted by Xu et al. 17 By analyzing time series CT scans, the deep learning algorithms could predict survival, progression, distant metastases, and local-regional recurrence. These studies illustrate the significant potential of AI in monitoring and predicting treatment responses.
AI applications can also assist clinicians in monitoring patients for potential toxicity. Many pre-clinical studies have deployed AI phenotyping algorithms to identify radiographic or EHR-based features predictive of cancer-therapy-related toxicity: notably, the SHIELD study by Hong et al.,18 a prospective clinical trial involving an EHR-based machine learning prediction algorithm in which patients undergoing outpatient radiotherapy or chemoradiation identified by machine learning as high risk (>10% risk of acute care during treatment) were referred for more intensive twice-weekly clinical evaluations. This significantly reduced acute care visits during treatment from 22.3% to 12.3%, thus demonstrating how machine learning can effectively triage patients and direct clinical management to enhance care quality and reduce healthcare costs.
As we have seen through these applications, AI has made significant strides in oncology. However, these current achievements only represent the tip of the iceberg when envisioning the future scope of AI in medicine.
Read part 2—Demystifying AI: How Will Artificial Intelligence Alter the Practice of Thoracic Oncology?
References
- 1. Bini SA. Artificial intelligence, machine learning, deep learning, and cognitive computing: What do these terms mean and how will they impact health care? J Arthroplasty. 2018;33(8):2358-2361. doi:10.1016/j.arth.2018.02.067
- 2. Helm JM, Swiergosz AM, Haeberle HS, et al. Machine learning and artificial intelligence: Definitions, applications, and future directions. Curr Rev Musculoskelet Med. 2020;13(1):69-76. doi:10.1007/s12178-020-09600-8
- 3. LeCun Y, Bengio Y, Hinton G. Deep learning. Nature. 2015;521(7553):436-444. doi:10.1038/nature14539
- 4. Raffel C, Shazeer N, Roberts A, et al. Exploring the limits of transfer learning with a unified text-to-text transformer. arXiv [csLG]. Published online October 23, 2019. http://arxiv.org/abs/1910.10683
- 5. Parikh RB, Manz C, Chivers C, et al. Machine learning approaches to predict 6-month mortality among patients with cancer. JAMA Netw Open. 2019;2(10):e1915997. doi:10.1001/jamanetworkopen.2019.15997
- 6. Manz CR, Chen J, Liu M, et al. Validation of a machine learning algorithm to predict 180-day mortality for outpatients with cancer. JAMA Oncol. 2020;6(11):1723-1730. doi:10.1001/jamaoncol.2020.4331
- 7. Hosny A, Bitterman DS, Guthier computer vision, et al. Clinical validation of deep learning algorithms for radiotherapy targeting of non-small-cell lung cancer: an observational study. Lancet Digit Health. 2022;4(9):e657-e666. doi:10.1016/S2589-7500(22)00129-7
- 8. Milam ME, Koo CW. The current status and future of FDA-approved artificial intelligence tools in chest radiology in the United States. Clin Radiol. 2023;78(2):115-122. doi:10.1016/j.crad.2022.08.135
- 9. Mikhael PG, Wohlwend J, Yala A, et al. Sybil: A validated deep learning model to predict future lung cancer risk from a single low-dose chest computed tomography. J Clin Oncol. 2023;41(12):2191-2200. doi:10.1200/JCO.22.01345
- 10. Lu MT, Raghu VK, Mayrhofer T, Aerts HJWL, Hoffmann U. Deep Learning Using Chest Radiographs to Identify High-Risk Smokers for Lung Cancer Screening Computed Tomography: Development and Validation of a Prediction Model. Ann Intern Med. 2020;173(9):704-713. doi:10.7326/M20-1868
- 11. Coudray N, Ocampo PS, Sakellaropoulos T, et al. Classification and mutation prediction from non-small cell lung cancer histopathology images using deep learning. Nat Med. 2018;24(10):1559-1567. doi:10.1038/s41591-018-0177-5
- 12. Chaunzwa TL, Hosny A, Xu Y, et al. Deep learning classification of lung cancer histology using CT images. Sci Rep. 2021;11(1):5471. doi:10.1038/s41598-021-84630-x
- 13. Vanguri RS, Luo J, Aukerman AT, et al. Multimodal integration of radiology, pathology and genomics for prediction of response to PD-(L)1 blockade in patients with non-small cell lung cancer. Nat Cancer. 2022;3(10):1151-1164. doi:10.1038/s43018-022-00416-8
- 14. Xu H, Mohamed M, Flannery M, et al. An unsupervised machine learning approach to evaluating the association of symptom clusters with adverse outcomes among older adults with advanced cancer: A secondary analysis of a randomized clinical Trial. JAMA Netw Open. 2023;6(3):e234198. doi:10.1001/jamanetworkopen.2023.4198
- 15. Kehl KL, Xu W, Gusev A, et al. Artificial intelligence-aided clinical annotation of a large multi-cancer genomic dataset. Nat Commun. 2021;12(1):7304. doi:10.1038/s41467-021-27358-6
- 16. Kehl KL, Elmarakeby H, Nishino M, et al. Assessment of deep natural language processing in ascertaining oncologic outcomes from radiology reports. JAMA Oncol. 2019;5(10):1421-1429. doi:10.1001/jamaoncol.2019.1800
- 17. Xu Y, Hosny A, Zeleznik R, et al. Deep learning predicts lung cancer treatment response from serial medical imaging. Clin Cancer Res. 2019;25(11):3266-3275. doi:10.1158/1078-0432.ccr-18-2495
- 18. Hong JC, Eclov NCW, Dalal NH, et al. System for high-intensity evaluation during radiation therapy (SHIELD-RT): A prospective randomized study of machine learning-directed clinical evaluations during radiation and chemoradiation. J Clin Oncol. 2020;38(31):3652-3661. doi:10.1200/JCO.20.01688