Author’s Note: Our previous article, Demystifying AI: Current Insights on Artificial Intelligence in Thoracic Oncology, reviews the foundational principles of AI, AI methods, and the role of AI in thoracic oncology, laying the groundwork for discussions here and those that follow in part 2 in the coming weeks.
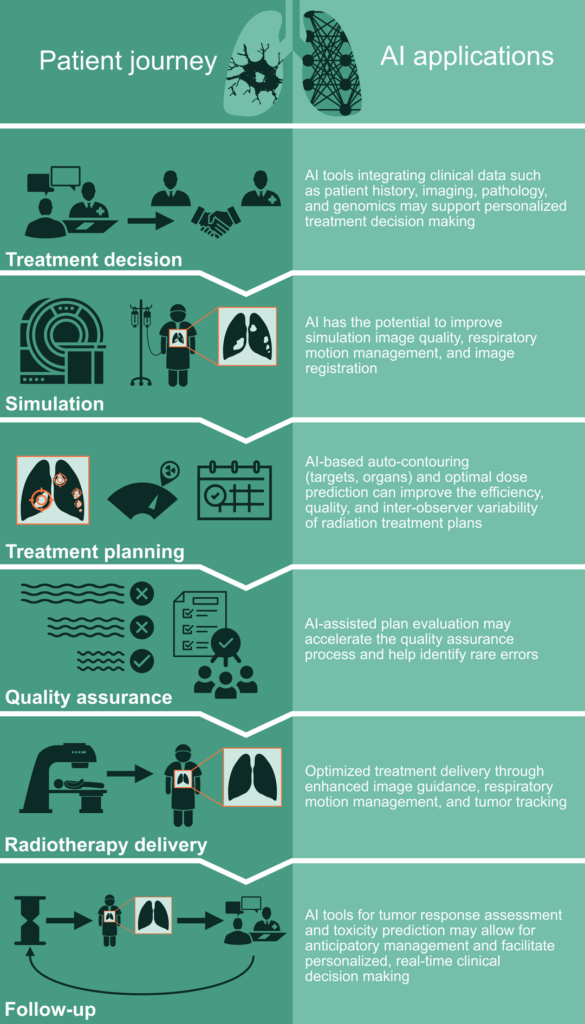
Radiation therapy (RT) is a critical treatment modality for lung cancer. Indications for RT in thoracic oncology include definitive treatment, preoperative or postoperative therapy, and palliative treatment.1,2 Various RT techniques are used such as stereotactic ablative RT for early stage (I-II) non-small cell lung cancer (NSCLC), long-course conventionally fractionated RT with concurrent chemotherapy for locally advanced (III) NSCLC and limited stage (I-III) small cell lung cancer, and palliative RT for stage IV lung cancer.3 Planning and delivery of RT for lung cancer patients rely on human-machine interaction including both software and hardware. With the growing role of artificial intelligence (AI) in transforming various fields in medicine, AI also has the potential to enhance the workflow of radiation oncology and improve the quality of care. In this article, we review the recent advances and potential role of AI in thoracic radiation oncology (Figure 1).
Patient Evaluation and Treatment Decision-Making
AI methods such as medical imaging analysis and natural language processing (NLP) based on electronic medical records (EMR) can facilitate automatic aggregation and interpretation of relevant clinical data to provide decision support for final treatment plans. AI in radiation oncology can also be used to predict histology based on imaging, determine, radiosensitivity of tumors based on CT-derived features,4,5 and gauge early tumor response prediction to chemoradiotherapy based on sequential PET/CT images6 Deep learning networks based on CT images have demonstrated superior mortality risk stratification compared to prediction models based on clinical parameters in NSCLC patients.7 Moreover, deep learning-based automated body composition measures8 and coronary artery calcium quantification9 based on CT images have been shown to be predictive of survival in the lung cancer population.
Treatment Planning
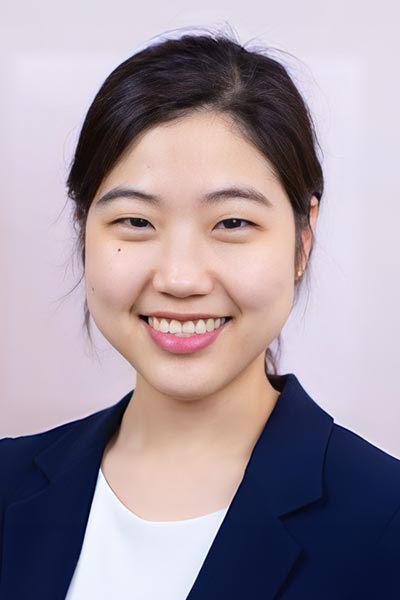
RT planning is a complex process heavily dependent on human-machine interaction. It entails image-based “simulation” of the patient, contouring of target volumes and organs, design of the radiation treatment plan, and the quality assurance check with collaboration of the radiation therapist, medical dosimetrist, medical physicist, and radiation oncologist at various steps. Since each step of the RT planning process is already completely digitized, there is significant potential for AI tools to enhance the efficiency, accuracy, and quality of lung cancer RT planning.
Simulation
RT simulation for lung cancer patients includes immobilization and CT-image acquisition often in 4-dimensions (4D) to capture the respiratory motion. AI can be leveraged to help predict the most effective simulation approach for each lung cancer patient based on their unique anatomical and clinical profile derived from diagnostic imaging and relevant history. Following simulation, acquired CT images are often registered with diagnostic imaging such as PET scans to enhance tumor delineation. However, image registration can be imprecise because of differences in patient orientation or position, motion, and/or image artifacts. In such circumstances, AI tools have the potential to improve image registration across different image modalities and artifacts.10,11,12,13
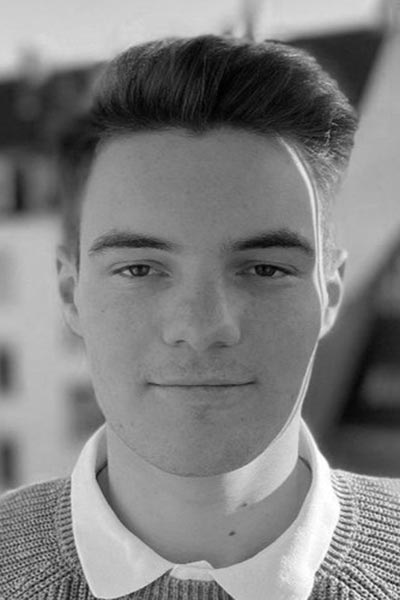
Contouring
Manual contouring or delineation of target and nearby organ volumes is a critical step in RT planning. However, it is also a time-consuming and labor-intensive process. Significant inter-observer variations also exist between different clinicians and institutions, which can result in disparities in treatment quality and outcomes. In recent years, AI-driven auto-contouring tools that parallel human experts’ contouring abilities have been developed and integrated into the radiation oncology workflow, leading to significant gains in efficiency.14,15 Advanced organ delineation systems such as the TotalSegmentator also provide granular body segmentation including lobar level segmentations in the lung and cardiac sub-structures.16 Auto-contouring tools not only improve efficiency but may also allow for reduction of inter-observer variability17,18 and inclusion of many other organ substructures such as the left anterior descending artery in lung cancer treatment, which is key for optimization of dose planning to reduce toxicity.
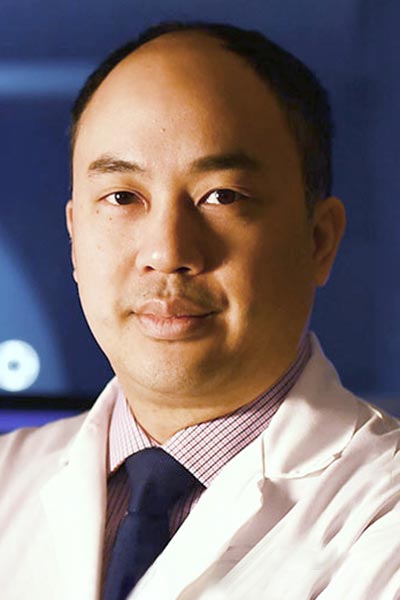
Design of the Radiation Treatment Plan
Once contours are finalized, medical dosimetrists design the RT plan based on the radiation oncologist’s treatment intent. This process entails selection of beam angles and optimization parameters followed by a series of fine modifications to achieve an optimal plan meeting pre-specified goals for target coverage and organ dose constraints. This is a time-consuming process and can result in significant variability in plan quality.19,20 Automated treatment planning can aid in: 1) prediction of optimal dose distribution and 2) selection of machine parameters and beam orientation to achieve such dose distribution.21,22,23 While still at early stages in development, the perfection of such methods may allow for fully, automated treatment planning.
Quality Assurance
The final step in RT planning is quality assurance by the medical physicist, which currently includes manual checks of plan parameters and physical measurements of radiation dose delivery. AI tools could accelerate this process and help identify rare errors.24 AI algorithms can predict quality assurance passing rates based on the RT plan itself and detect potential sources of error, without the need for physical dose measurements.25 Harnessing data accumulated from numerous quality assurance evaluations, AI algorithms may also aid in predicting machine errors.26,27
References
- 1. Ettinger DS, Wood DE, Aisner DL, et al. NCCN Guidelines® Insights: Non–Small Cell Lung Cancer, Version 2.2023: Featured Updates to the NCCN Guidelines. Journal of the National Comprehensive Cancer Network. 2023;21(4):340-350. doi:10.6004/jnccn.2023.0020
- 2. Ganti AKP, Loo BW, Bassetti M, et al. Small Cell Lung Cancer, Version 2.2022, NCCN Clinical Practice Guidelines in Oncology. Journal of the National Comprehensive Cancer Network. 2021;19(12):1441-1464. doi:10.6004/jnccn.2021.0058
- 3. Vinod SK, Hau E. Radiotherapy treatment for lung cancer: Current status and future directions. Respirology. 2020;25(S2):61-71. doi:10.1111/resp.13870
- 4. Chaunzwa TL, Hosny A, Xu Y, et al. Deep learning classification of lung cancer histology using CT images. Sci Rep. 2021;11(1):5471. doi:10.1038/s41598-021-84630-x
- 5. Lou B, Doken S, Zhuang T, et al. An image-based deep learning framework for individualizing radiotherapy dose: a retrospective analysis of outcome prediction. The Lancet Digital Health. 2019;1(3):e136-e147. doi:10.1016/S2589-7500(19)30058-5
- 6. Buizza G, Toma-Dasu I, Lazzeroni M, et al. Early tumor response prediction for lung cancer patients using novel longitudinal pattern features from sequential PET/CT image scans. Phys Med. 2018;54:21-29. doi:10.1016/j.ejmp.2018.09.003
- 7. Hosny A, Parmar C, Coroller TP, et al. Deep learning for lung cancer prognostication: A retrospective multi-cohort radiomics study. Butte AJ, ed. PLoS Med. 2018;15(11):e1002711. doi:10.1371/journal.pmed.1002711
- 8. Al-Sawaf O, Weiss J, Skrzypski M, et al. Body composition and lung cancer-associated cachexia in TRACERx. Nat Med. 2023;29(4):846-858. doi:10.1038/s41591-023-02232-8
- 9. Atkins KM, Weiss J, Zeleznik R, et al. Elevated Coronary Artery Calcium Quantified by a Validated Deep Learning Model From Lung Cancer Radiotherapy Planning Scans Predicts Mortality. JCO Clinical Cancer Informatics. 2022;(6):e2100095. doi:10.1200/CCI.21.00095
- 10. Miao S, Wang ZJ, Liao R. Real-time 2D/3D Registration via CNN Regression. Published online 2015. doi:10.48550/ARXIV.1507.07505
- 11. Miao, S., Piat, S., Fischer, P., Tuysuzoglu, A., Mewes, P., Mansi, T. and Liao, R. Dilated FCN for Multi-Agent 2D/3D Medical Image Registration. in (2017).
- 12. Ma, K. et al. Multimodal Image Registration with Deep Context Reinforcement Learning. in Lecture Notes in Computer Science 240–248 (2017).
- 13. Hou B, Alansary A, McDonagh S, et al. Predicting Slice-to-Volume Transformation in Presence of Arbitrary Subject Motion. Published online 2017. doi:10.48550/ARXIV.1702.08891
- 14. Lustberg T, van Soest J, Gooding M, et al. Clinical evaluation of atlas and deep learning based automatic contouring for lung cancer. Radiother Oncol. 2018;126(2):312-317. doi:10.1016/j.radonc.2017.11.012
- 15. Mak RH, Endres MG, Paik JH, et al. Use of Crowd Innovation to Develop an Artificial Intelligence-Based Solution for Radiation Therapy Targeting. JAMA Oncol. 2019;5(5):654-661. doi:10.1001/jamaoncol.2019.0159
- 16. Wasserthal J, Breit HC, Meyer MT, et al. TotalSegmentator: Robust Segmentation of 104 Anatomic Structures in CT Images. Radiology: Artificial Intelligence. 2023;5(5):e230024. doi:10.1148/ryai.230024
- 17. Vinod SK, Min M, Jameson MG, Holloway LC. A review of interventions to reduce inter-observer variability in volume delineation in radiation oncology. J Med Imaging Radiat Oncol. 2016;60(3):393-406. doi:10.1111/1754-9485.12462
- 18. Hosny A, Bitterman DS, Guthier CV, et al. Clinical validation of deep learning algorithms for radiotherapy targeting of non-small-cell lung cancer: an observational study. The Lancet Digital Health. 2022;4(9):e657-e666. doi:10.1016/S2589-7500(22)00129-7
- 19. Nelms BE, Robinson G, Markham J, et al. Variation in external beam treatment plan quality: An inter-institutional study of planners and planning systems. Pract Radiat Oncol. 2012;2(4):296-305. doi:10.1016/j.prro.2011.11.012
- 20. Berry SL, Boczkowski A, Ma R, Mechalakos J, Hunt M. Interobserver variability in radiation therapy plan output: Results of a single-institution study. Pract Radiat Oncol. 2016;6(6):442-449. doi:10.1016/j.prro.2016.04.005
- 21. Barragán-Montero AM, Nguyen D, Lu W, et al. Three-dimensional dose prediction for lung IMRT patients with deep neural networks: robust learning from heterogeneous beam configurations. Med Phys. 2019;46(8):3679-3691. doi:10.1002/mp.13597
- 22. Fan J, Wang J, Chen Z, Hu C, Zhang Z, Hu W. Automatic treatment planning based on three‐dimensional dose distribution predicted from deep learning technique. Medical Physics. 2019;46(1):370-381. doi:10.1002/mp.13271
- 23. Tseng HH, Luo Y, Cui S, Chien JT, Ten Haken RK, Naqa IE. Deep reinforcement learning for automated radiation adaptation in lung cancer. Med Phys. 2017;44(12):6690-6705. doi:10.1002/mp.12625
- 24. Kalendralis P, Luk SMH, Canters R, et al. Automatic quality assurance of radiotherapy treatment plans using Bayesian networks: A multi-institutional study. Front Oncol. 2023;13:1099994. doi:10.3389/fonc.2023.1099994
- 25. Valdes G, Chan MF, Lim SB, Scheuermann R, Deasy JO, Solberg TD. IMRT QA using machine learning: A multi-institutional validation. J Appl Clin Med Phys. 2017;18(5):279-284. doi:10.1002/acm2.12161
- 26. Carlson JNK, Park JM, Park SY, Park JI, Choi Y, Ye SJ. A machine learning approach to the accurate prediction of multi-leaf collimator positional errors. Phys Med Biol. 2016;61(6):2514-2531. doi:10.1088/0031-9155/61/6/2514
- 27. Li Q, Chan MF. Predictive time-series modeling using artificial neural networks for Linac beam symmetry: an empirical study. Ann N Y Acad Sci. 2017;1387(1):84-94. doi:10.1111/nyas.13215
- 28. Kida S, Nakamoto T, Nakano M, et al. Cone Beam Computed Tomography Image Quality Improvement Using a Deep Convolutional Neural Network. Cureus. 2018;10(4):e2548. doi:10.7759/cureus.2548
- 29. Isaksson M, Jalden J, Murphy MJ. On using an adaptive neural network to predict lung tumor motion during respiration for radiotherapy applications. Med Phys. 2005;32(12):3801-3809. doi:10.1118/1.2134958