CT screening for lung cancer can reveal subtle early signs of disease in the lungs that extend beyond potential malignancy. As reviewed by Bram van Ginneken, PhD, of the Radboud University Medical Center in The Netherlands, quantitative measures obtained from a chest CT scan may enable the early detection of different obstructive and interstitial lung diseases, such as chronic obstructive pulmonary disease (COPD) and idiopathic pulmonary fibrosis (IPF), while also informing the optimal frequency of lung cancer screening. Moreover, many of these measures can be automated using artificial intelligence (AI).
Emphysema Measures
The quantification of emphysema on CT images is a well-established technique in which computerized methods are applied to determine the percentage of lung voxels that fall below a certain threshold, yielding an emphysema score. However, there are some drawbacks to this approach, which, until recently, limited its clinical applicability.
A key shortcoming of the emphysema score involves heterogeneous image quality—for example, whether an image features a soft or sharp reconstruction kernel—which can dramatically alter the score. To overcome this limitation, Dr. van Ginneken and colleagues developed a standardization technique that basically re-renders all CT images with the same reconstruction kernel (Fig. 1).1
Applying this correction factor to the images improves the ability of the emphysema score to predict all-cause and lung-cancer mortality in patients at high-risk for lung cancer. It also enables the emphysema score to serve as a CT biomarker, signaling when to potentially initiate early treatment for emphysema or apply more frequent screening.
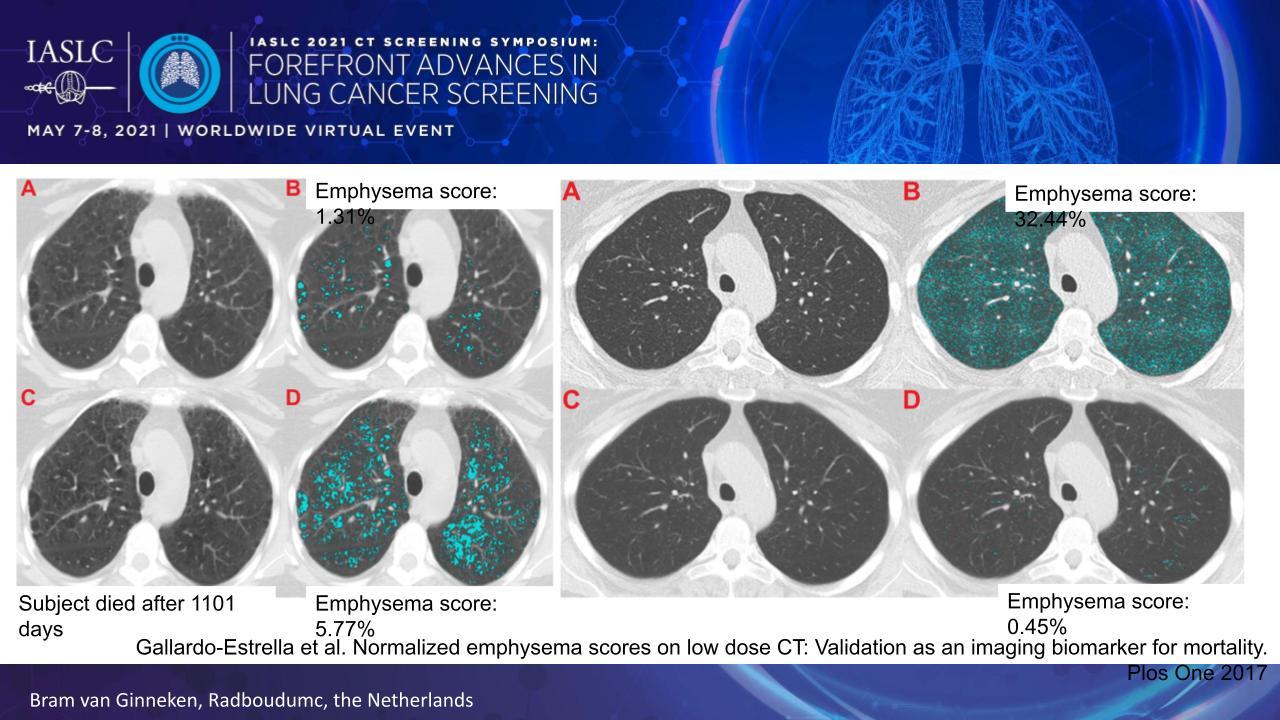
Another drawback of a simple threshold-based emphysema score is that it does not account for the type of emphysema, of which there are now 3 major types and 7 additional subtypes based on CT imaging patterns. Very recently, researchers devised an AI solution in which they trained a computer algorithm to grade emphysema in accord with the CT-definable subtypes, and they demonstrated that the resulting grades correlated with clinical measures of pulmonary insufficiency and the risk of mortality.2
Airway Measures
Obstructive airway disease can be quantified by measuring the thickness of the airway walls. Collecting these measures at multiple locations throughout the airway tree generates a data set that allows one to determine the average wall thickness for an airway with a 10-mm lumen perimeter on CT, referred to as the Pi10 (Fig. 2).
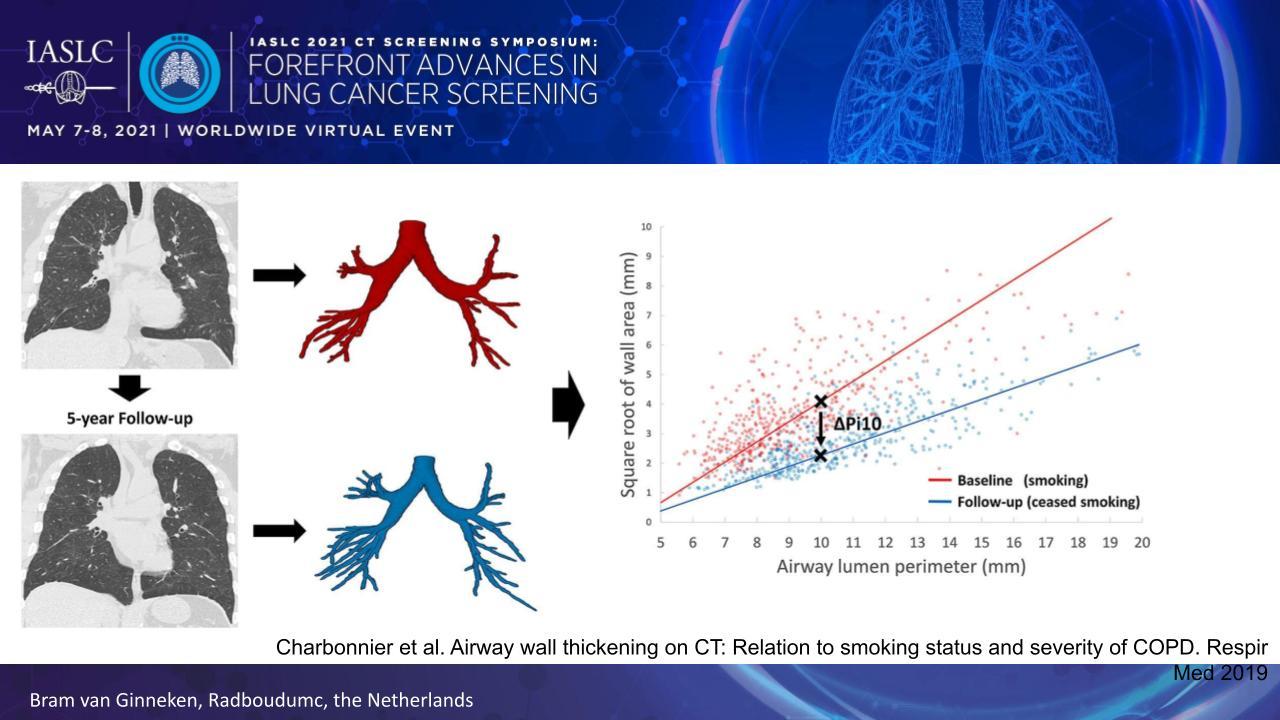
The Pi10 is useful because it correlates with smoking status and COPD severity.3
In addition, the Pi10 demonstrates the ability to predict which individuals with COPD will experience a clinically meaningful improvement in FEV1 with treatment.4
Unfortunately, the Pi10 does not perform particularly well in predicting treatment response, as illustrated by an area under the receiver operating characteristic (ROC) curve of only 0.641.
“This means that in the context of lung cancer screening, if you would want to use this measurement to decide in whom to start on treatment for COPD, it’s not a very good model yet,” Dr. van Ginneken remarked.
Interstitial Lung Abnormality Measures
“Interstitial lung abnormalities can be a precursor to the development of IPF, and clinically diagnosed IPF is associated with a very poor survival, actually comparable to lung cancer. It would be interesting to see if we could find [these abnormalities] in an early stage in subjects that are participating in lung cancer screening,” Dr. van Ginneken said.
Indeed, studies have shown that interstitial lung abnormalities can be identified in 10% to 17% of all lung cancer screening CT scans.5
,6
With continued follow-up, nonfibrotic and fibrotic lesions demonstrate progression in 12% to 37% of patients, respectively, at 2 years, and they correlate with a higher risk of subsequent diagnosis of interstitial lung disease, COPD, pneumonia, lung cancer, and respiratory failure.5
,6
Automated algorithms have been developed to identify and classify interstitial lung abnormality patterns in CT images. These algorithms demonstrate impressive performance, suggesting that clinicians can put AI to work to identify radiographic patterns that precede the development of IPF.7
However, with all early interstitial and obstructive lung diseases that may be detected on lung cancer screening CT scans, Dr. van Ginneken stressed the need to have a plan in place in the event of a positive finding. “If you do not know what to do when you see that early sign, then it might not be relevant to detect it in the first place,” he remarked.
Risk Modeling and Personalized Screening
Beyond the early detection of other lung diseases, quantitated CT scan measures can be used to decide when to perform follow-up lung cancer screening scans in individual patients. Researchers have built risk models that include a wide variety of variables—age, nodule features, mean lung density, emphysema score, bronchial wall thickness, and aorta calcium volume—the majority of which are derived from AI-driven quantification of the CT scans.8
These models prove to be fairly accurate at determining which individuals require annual lung cancer screening and which are safe to undergo biennial screening with little risk of a delayed diagnosis.
Dr. van Ginneken explained that applying these risk models opens the door to more lenient enrollment criteria for screening programs. “Allow more people to get a baseline CT scan, and then use the information from the CT scans to decide when to perform a next scan,” he suggested.
- 1. Gallardo-Estrella L, Pompe E, de Jong PA, et al. Normalized emphysema scores on low dose CT: Validation as an imaging biomarker for mortality. PLoS One. 2017;12(12):e0188902.
- 2. Humphries SM, Notary AM, Centeno JP, et al; Genetic Epidemiology of COPD (COPDGene) Investigators. Deep learning enables automatic classification of emphysema pattern at CT. Radiology. 2020;294(2):434-444.
- 3. Charbonnier JP, Pompe E, Moore C, et al; COPDGene investigators. Airway wall thickening on CT: Relation to smoking status and severity of COPD. Respir Med. 2019;146:36-41.
- 4. Park HJ, Lee SM, Choe J, et al. Prediction of treatment response in patients with chronic obstructive pulmonary disease by determination of airway dimensions with baseline computed tomography. Korean J Radiol. 2019;20(2):304-312.
- 5. a. b. Jin GY, Lynch D, Chawla A, et al. Interstitial lung abnormalities in a CT lung cancer screening population: prevalence and progression rate. Radiology. 2013;268(2):563-571.
- 6. a. b. Hoyer N, Thomsen LH, Wille MMW, et al. Increased respiratory morbidity in individuals with interstitial lung abnormalities. BMC Pulm Med. 2020;20(1):67.
- 7. Bermejo-Peláez D, Ash SY, Washko GR, San José Estépar R, Ledesma-Carbayo MJ. Classification of interstitial lung abnormality patterns with an ensemble of deep convolutional neural networks. Sci Rep. 2020;10(1):338.
- 8. Schreuder A, Jacobs C, Lessmann N, et al. Combining pulmonary and cardiac computed tomography biomarkers for disease-specific risk modelling in lung cancer screening. Eur Respir J. 2021:2003386.